FROM PROF. DR. PETER GENTSCH
The AI framework enables the bridging of the infrastructure via the use cases to the business.
AI FRAMEWORK – THE 360° PERSPECTIVE
Within the AI Business Framework, the relevant topics and terms are systematized, classified and linked to each other. The AI Framework thus acts as a transmission belt from the success factors and drivers of AI in companies to the business applications. The AI Business Framework shows the entire range of tools and solutions and is thus intended to provide better orientation in the AI jungle. It is not possible to always clearly assign data, technologies, methods, use cases and business applications. The relationships are far too complex and multi-layered.
LAYERS OF THE AI FRAMEWORK
The success factors of AI form the lowest layer, the so-called enabler layer. Thus, due to its contribution to the development of AI and the emergence of Big Data, web technologies, multi-core processors, distributed systems, GPUs, as well as future technologies and synapse and quantum chips were incorporated into the framework. The significance of Big Data for the current development of artificial intelligence is taken into account with a separate layer.
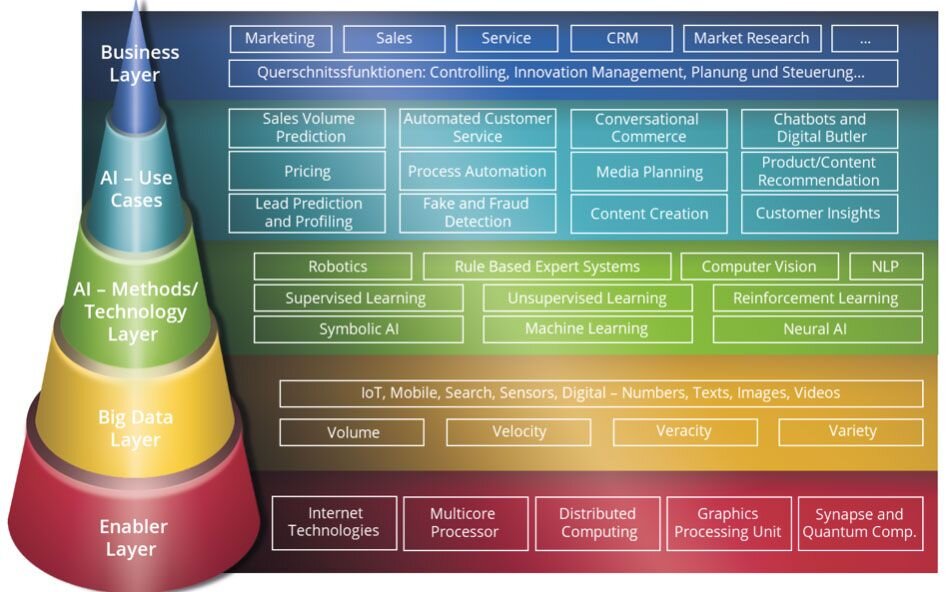
Fig. Business AI Framework
Within this layer, special attention is paid to the following points:
Structured and unstructured data (Variety). Methods derived from AI research allow the analysis of structured and unstructured data.
Large amounts of data for the training of machine learning algorithms (Volume) are crucial for AI developments.
The speed (velocity) in combination with the data volumes used to generate and evaluate data can no longer be handled by human actors without the support of intelligent systems. ML algorithms help to control the flood of data and to separate the important from the unimportant.
The credibility of the data (veracity) is also difficult to determine manually. Currently, systems are being worked on that are supposed to distinguish real news from fake news
As a separate item, data sources are shown in the framework: whether from the Internet of Things (IoT), mobile devices, search applications, sensors or other digital applications. Data is the fuel for the AI machine. Neither its origin nor its structure is decisive, nor can it become “too much” data nowadays.
AI USE CASES
For the layer that unites the business and AI worlds, the “Artificial Intelligence Use Cases Layer”, a multitude of present and future examples can be found. In the following, the Use Cases are presented and explained as a further layer for the AI Business Framework.
AUTOMATED CUSTOMER SERVICE
In conjunction with the development of personal assistants, advances in computational linguistics have also made it possible to make companies’ customer service departments much more efficient. While the customer experience with calls is often negative nowadays due to answers such as “Unfortunately, your answer was not understood, did you mean…?”, NLP algorithms help to make such experiences a thing of the past and to ensure that simple requests can actually be clarified comfortably in natural language.
CONTENT CREATION
Content marketing and target group-relevant addressing have been preached as a formula for success in marketing for quite some time. However, the potential of digitally available data for automated content creation is usually not used. For example, algorithms can automatically generate interesting and unadulterated insights in real time based on public Internet data. For example, infographics can be generated automatically to show business development for selected industries depending on the use of certain technologies, the level of digital maturity or the use of advertising. Likewise, new market developments and emerging issues can be automatically identified on the basis of Big Data from the Internet.
In this way, current discussions and reports can be used systematically (“news jacking”). The editorial description and explanation of the insights generated is carried out by an appropriate analysis team. Computational linguistics, more precisely the Natural Language Generation, is used for this purpose. This refers to systems that create texts based on numbers and individual facts. These are difficult to distinguish from human written texts. Due to their consistent structure, sports or financial news are particularly suitable for this purpose.
CONVERSATIONAL COMMERCE, CHAT BOTS AND PERSONAL ASSISTANTS
Instead of artificial interfaces such as websites and apps, customers can communicate with corporate systems via natural communication in the sense of spoken or written language. This is made possible by the developments in computational linguistics described above. This type of communication also enables people with less technical affinity to use new technologies – currently, various providers are competing for the best personal assistants.
And with good reason: companies that can assert themselves here and sell their solutions to customers will in the medium term develop into a kind of portal for other companies to sell their products to customers. This is why this topic is currently very high on the agendas of companies such as Amazon, Apple or Google.
CUSTOMER INSIGHTS
One of the central tasks of classical market research is the systematic derivation and explanation of “how the customer ticks” – known as customer insight. In order to obtain the assessment of customers about products, classical market research has a comprehensive range of instruments at its disposal: focus groups, customer surveys, panels, etc. The main disadvantage of this primary research is the expense involved. On the Internet, for example, thousands of product evaluations can be analyzed automatically at any time: Ratings and reviews, which are globally distributed over various Internet platforms, are intelligently recorded and integrated via bots.
With the help of NLP, the central customer statements are automatically extracted from the free texts of the reviews. In order to gain deeper insights, the insights gained must be correlated with other data such as complaints, sales or customer satisfaction.
FAKE AND FRAUD DETECTION
AI has been used for some time in classical fraud detection and prediction (Fraud Detection). In the field of marketing and communication there is currently talk of fake news and manipulation through targeted disinformation. The use of (chat) bots for targeted promotion, disinformation and manipulation carries a high risk for companies. However, the topic is not new. In the past, many companies have commissioned agencies to remove or cover up negative posts in social networks, to push topics or to give positive ratings or negative ratings for the competitor.
Some companies have not survived exposure, or have suffered image damage or have had to endure severe shit storms. We have also been dealing with the phenomenon of astroturfing for a long time. Now this process can be automated and scaled. Algorithmics and AI can also help here. A systematic, data-driven approach can automatically detect patterns of manipulative bots, such as posting frequency and times, network of followers, content and tonalities. Modern AI methods are used for detection and prevention. These methods have also been successfully used for click and credit card fraud. In a sense, we beat the manipulative bots with the same weapons they use for automated disinformation and manipulation.
LEAD PREDICTION AND PROFILING
AI enables the automated recognition and profiling of potential customers. For example, new customers and markets can be identified and characterized on the basis of given customer profiles via so-called statistical twins. In the process, the selected companies were condensed with thousands of attributes into a digital signature. Based on these data vectors, new customers can be predicted in the digital space using AI algorithms (predictive analytics). In this way, leads and markets can be identified that are not part of the classic prey scheme, but represent potential buyers – communication potential beyond the traditional industry and segment view. Dynamic profiling can also automatically identify and evaluate communication and sales triggers: At which event is the sales approach particularly successful? Time- and context-specific sales signals significantly increase the probability of conversion. In addition, the trigger can also be used as a communication trigger for the concrete sales approach. In addition to the addresses of the companies, it is also possible to provide indications for the right communication approach: If necessary, a direct approach to Xing and LinkedIn is more promising than a phone call or e-mail.
MEDIA PLANNING
For years, the media market has been politically shaped by selfish and interest-driven projects and arguments. Algorithm-based technology platforms enable transparent and efficient media planning based on artificial intelligence. AI and algorithms can capture a multitude of relevant active and reactive media data points and systematically evaluate them automatically. Thus, the so often subjective and self-interest driven planning experiences an empirical grounding and validation.
PRICING
The use of AI software to determine retail prices for everything from gasoline to office supplies to food is growing. It’s not about how competition changes prices. AI algorithms analyze thousands of data points on an ongoing basis and calculate prices that the software believes consumers are willing to pay. In other words, it’s about finding the optimal price – not the lowest. AI pricing software analyzes vast amounts of historical and real-time data and attempts to determine how consumers will react to a price change under certain scenarios. Tactics are updated based on experience. Likewise, these solutions try to learn and take into account human behavior. Overall, it is not about getting more money out of customers in general. Rather, it is about making margins with customers who don’t care and not making margins with customers who care. The use of AI pricing algorithms is growing steadily in Europe and in the USA, especially for gas stations. The approach is particularly exciting for retailers. Staples, for example, uses AI to set prices of more than 30,000 products on their websites every day. The mother of all online retailers in the USA, Amazon and its third-party vendors, was among the first to use dynamic pricing, a precursor to AI pricing. Today, Amazon makes extensive use of algorithmic AI technologies to maximize consumer surplus.
PROCESS AUTOMATION
The topic of process automation is not new. It was intensively discussed and implemented in the 1990s as part of the so-called Business Process Management/Reengineering. The focus was more on industrial and manufacturing processes and less on marketing and sales processes. Moreover, algorithmic support was mostly classically rule-based. Robotic Process Automation (RPA) is a software automation tool that automates routine tasks such as data extraction and preparation. The robot has a kind of user ID like a person and can perform rule-based tasks such as accessing e-mail and other systems, calculating calculations, creating documents and reports, and checking files. For example, RPA helped a large insurance company reduce queuing procedures that affected 2500 high-risk accounts per day. This helped reduce the workload on 81% of the staff, who could then focus on proactive account management. Thanks to modern AI algorithms, intelligent process automation can achieve significantly improved efficiency, increased employee performance, reduced operational risks, improved response times and an optimized customer experience.
PRODUCT/CONTENT RECOMMENDATION
Recommendations for products and/or content are often proposed and maintained manually by editors and shop managers. However, this is very time-consuming and scales poorly. Recommendation engines for personalized recommendations are indispensable in a modern webshop today. While in the early days simple algorithms for shopping basket analyses were implemented – “Customers who bought product A also bought product B” – today, AI procedures that take a large number of data points into account are increasingly being used. For example, based on their click and purchase behavior, users are shown additional suitable content to better satisfy their interest and create additional purchase incentives. A particularly promising approach is based on AI Reinforcement Learning. The open source approach of the GAFA world is also interesting here. Similar to how Google made the AI Deep Learning Framework Tensorflow public, Amazon is launching DSSTNE (pronounced “Destiny”) in 2016 as an open source AI framework that companies can use for their product recommendations. It may at first seem surprising that the “inventor” of automated product recommendation makes its core asset available to the community. But the rationale is clear, according to the corresponding Q&A section of Amazon: “We hope that researchers around the world will work together to further improve the recommendation system. But more importantly, we hope it will trigger innovation in many other areas”.
SALES VOLUME PREDICTION
The revenue forecasting process is critical for most companies, but it is also a difficult area of management. Most researchers and companies use statistical methods such as regression analysis to predict and analyze sales volumes. In addition, only a small amount of data is usually taken into account for forecasting sales. To improve the quality of the sales forecast, AI can take numerous other data points into account. These include historical as well as real time data, internal as well as external data, economic as well as environmental data, company-specific as well as micro- and macroeconomic data points (sales figures, stock data, prices, weather, holiday constellation, prices of competitors, etc.) Algorithms and AI help on the one hand to systematically and automatically extract these many structured and unstructured data points, and on the other hand, also automatically analyze them for accurate forecasting. One of the best known systems in Germany is the Blue Yonder solution.